Artificial Intelligence and Early Detection of Pancreatic Cancer
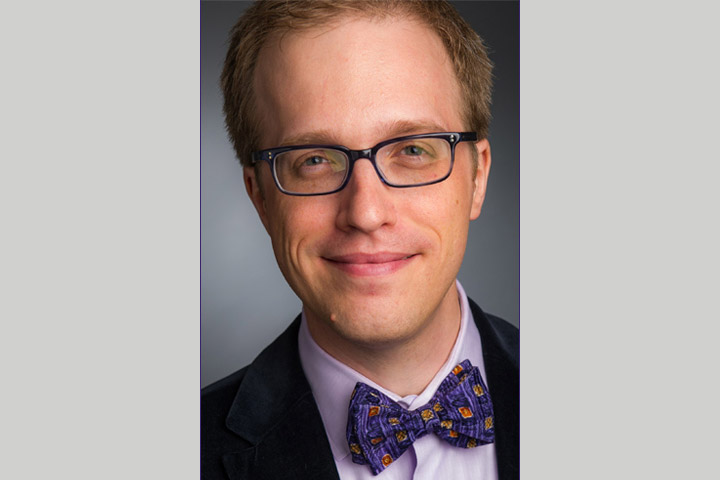
Artificial intelligence (AI) is no longer the stuff of science fiction.
Use a ridesharing app? That’s powered by AI. How about mobile check deposits? That’s powered by AI too. Even your email spam filter uses AI, though it’s clear sometimes important information winds up in your spam folder for no discernible reason.
The real promise of AI for those with pancreatic cancer lies in its astounding ability to digest and parse huge amounts of data and identify patterns that can elude even the most skilled physicians. Taken together, these AI attributes could potentially pave the way for earlier detection for a disease that is often diagnosed too late for potentially curative surgery. However, finding ways to tap into the full potential of AI requires skill sets spanning medicine, computer science, engineering, mathematics, industry, government, and more.
Gaining a better understanding of the current state of AI and where the science needs to go to achieve earlier detection was the focus of the AI and Early Detection of Pancreatic Cancer Virtual Summit, presented by Kenner Family Research Fund (KFRF) and the American Pancreatic Association.
The Summit brought together experts in AI to discuss current efforts and challenges in early detection. Subject areas discussed included the progress, problems, and prospects of early detection, AI and machine learning, current efforts in AI and early detection, collaborative opportunities, and insights from government, industry, and advocacy groups.
The goal of the Summit was to combine these various areas of expertise to develop a risk stratification model for early detection of pancreatic cancer using AI and machine learning, explains Barbara Kenner, Ph.D., founder of KFRF and an advocate for the National Cancer Institute’s Alliance of Pancreatic Cancer Consortia, the Pancreatic Cancer Detection Consortium (sponsored by the National Institutes of Health), and the Pancreatic Cancer Action Network (PanCAN) Early Detection Initiative, among others.
“Given that AI and machine learning are more frequently being applied in health care, we decided it was time to convene a summit that would provide an overview of the current AI landscape, and we wanted AI people involved outside the field of pancreatic cancer as well as those involved in pancreatic cancer detection and treatment to look at the space and help us understand what is needed to achieve the goal of earlier detection,” Kenner says. “The Summit was quite successful at achieving this, and now it’s on to the next steps.”
Leveraging Every Possible Tool
Those next steps can’t come soon enough for physician-scientist Michael Rosenthal, M.D., Ph.D., a diagnostic radiologist at Dana-Farber Cancer Institute and Brigham and Women’s Hospital, and an Assistant Professor of Radiology at Harvard Medical School (all in Boston, Massachusetts). Rosenthal is a specialist in the imaging of gastrointestinal cancers with a focus on pancreatic cancers.
“I’m a radiologist and a scientist with a biased of view of computer science since that’s my Ph.D.,” says Rosenthal, who served as co-leader of the “Current Efforts” discussion at the AI and Early Detection summit. Rosenthal makes it clear that it’s not so much that pancreatic cancer is a great target for AI, “but rather we need every tool we can possibly get to better deal with this cancer.”
“AI is great at complexity and pancreas cancer is an extraordinarily complex disease,” he adds.
It’s especially complex in terms of earlier detection. Since early pancreas abnormalities can be subtle and some of these cancers can grow quickly, it’s tough to find the malignancies early compared to other organs, Rosenthal says. Plus, symptoms like jaundice and weight loss often appear once the tumor has grown and spread.
Given these difficulties, pancreatic cancer is the toughest major cancer to detect early. “That’s where AI can come into the picture,” he explains. “We need to advance really in two areas. The first is to better understand how we can use AI to find features of pancreas cancer, and it was very impressive to see how researchers are moving forward in that area. Then we need to ensure that we are using those AI tools in the right population.”
The Power of Algorithms
One approach is to use what is called deep learning, a kind of subset of AI that uses algorithms to find patterns in massive amounts of data. “Data doesn’t just mean numbers,” Rosenthal says. “It can be anything that we can feed into a computer to help us find those important patterns and potential trends that might tip you off that something is going on with a patient that may be linked to the development or early diagnosis of pancreatic cancer.”
At the Mayo Clinic, for example, researchers are building algorithms to systematically identify those at higher risk for pancreas cancer, such as those with a family history of the disease. Scientists at other sites will also look for genetic mutations as well as new onset diabetes. At Dana-Farber a research team is also developing risk assessment models by using deep learning analysis of clinical records and images gathered from large, diverse patient population datasets to identify individuals at high‐risk for pancreatic cancer in the general population. These risk models will lead to a practical tool that can identify patients who are at elevated risk for pancreatic cancer and should be enrolled in screening programs for disease prevention and early detection.
“I’m very excited about research that looks at risk factors leading to more intensive surveillance,” Rosenthal says. “Changes in body composition, blood biomarkers, and new onset diabetes could lead us to catching pancreatic cancer earlier. It’s hopefully AI systems that will help us gather the necessary data to make that happen.”
He envisions a time—hopefully in the not too distant future—in which routine lab work and a routine doctor’s visit can result in pancreatic cancer surveillance for some patients. “It might be a case in which a patient’s weight goes down a little bit, and there’s been a decline in muscle mass, and some lab values are changing.”
“All of that might not raise a flag when taken alone, but when taken together based on an algorithm we’re going to see an important pattern and say let’s check this out more and see if there’s something going on with the pancreas.”
Interpreting Images
AI would seem especially adept at interpreting images for subtle changes, changes that are too tough for even the most skilled of radiologists to see—at least at first. A subset of deep learning techniques mimics the visual system of the human brain and is the most robust method of AI used to interpret images.
Leveraging the power of deep learning is another AI research endeavor at Johns Hopkins called the Felix Project, named after a potion made famous in the Harry Potter books. Drink the potion and success is yours.
“Deep learning kind of mimics the way our brain works,” Rosenthal says. “There are layers of computer algorithms, all of which are interconnected. As one layer gets information, it will then send it to the next layer. So information is gradually synthesized and condensed. The algorithms come to conclusions the way we would. We continually analyze data. So the more good data it gets, the better it gets at analysis.” The goal of the Felix Project is to develop a software program that analyzes CT images and flags suspicious areas.
“As a radiologist I’m really excited about this and as a team I have to say this group, which is so diverse in terms of skill sets, is absolutely delightful,” Rosenthal says. “They might be first out of the box to get this into the clinical setting.”
The Next Steps
What was clear at the Summit was that AI could potentially prove useful in a broad range of early detection research, including that of blood-based biomarkers, genetic analysis, and the tumor microbiome, among others, Rosenthal says.
According to KFRF, a comprehensive pre-summit paper providing an overview of current efforts in the field, an analysis of gaps and ideas for innovation and opportunity, along with a white paper outlining summit conversations and next steps will help in the development of an actionable strategic plan, implementation of operational standards, research priorities, and first-phase initiatives.
“Pancreatic cancer is a horrible disease, and it makes me pretty angry,” Rosenthal says. “It doesn’t, at this point, have great options for cure, so we need earlier detection because that’s a patient’s best chance.”
“It’s a disease that we just have to keep hammering away on. And AI is one of those tools that can help us with that. What was clear is that everyone at the Summit is and will continue to be committed to finding ways to find this disease early, early enough to make a difference.”