Using AI to Create a Better Test to Predict Pancreatic Cancer Progression
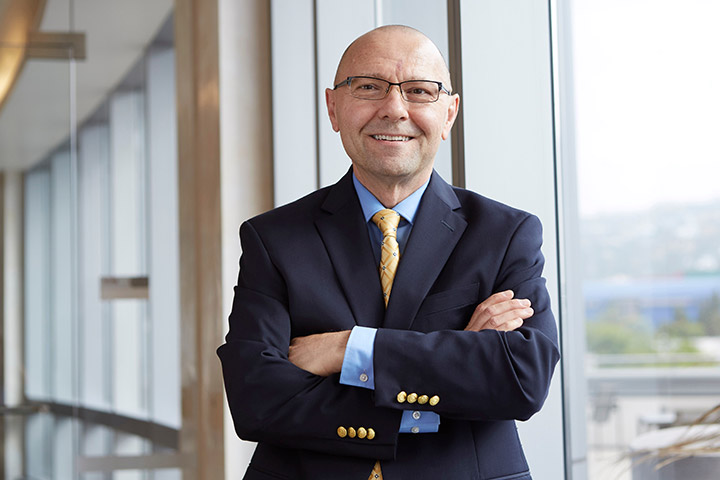
If you have pancreatic cancer, you are likely familiar with the CA 19-9 tumor marker blood test, which measures levels of tumor activity.
While it is somewhat useful in predicting pancreatic cancer progression, some people do not produce the antigen that the CA 19-9 test measures. What has long been needed is a better blood test for tracking the disease.
A research team led by Dan Theodorescu, M.D., Ph.D., the director of Cedars-Sinai Cancer in Los Angeles, California, is using artificial intelligence (AI) to do just that. In a study published in January 2024 in the journal Nature Cancer, Theodorescu’s team outlined an artificial intelligence and precision medicine approach, dubbed the Molecular Twin Precision Oncology Platform, MT-POP for short. The technology identified biomarkers that appear superior to the CA 19-9, the only Food and Drug Administration-approved pancreatic cancer test to predict pancreatic cancer survival.
The Significance of Precision Medicine
Back in 2015, then President Barack Obama announced the launch of the Precision Medicine Initiative (PMI). Nearly a decade later that innovative research effort has changed how many diseases are treated, especially cancer. Today, patients with breast or lung cancer, or melanoma, have benefitted greatly from a precision medicine approach. Advancements are also being made in pancreatic cancer as scientists gain a better understanding of the complex biology inherent to the disease.
Even when he was a child growing up in Romania, the complex biology of cancer fascinated Theodorescu, also the PHASE ONE Foundation Distinguished Chair at Cedars-Sinai. “I knew from a very young age that I wanted to do cancer research so I learned everything I could,” he says. “I read and watched documentaries and developed a real love of medicine, math, science, and technology.” For a disease like pancreatic cancer, as well as other highly lethal malignancies, unraveling the mysteries of its biology by bringing together experts from many different disciplines will ultimately lead to better treatments. And, equally as important, it will lead to a more rapid determination of which treatments work best for each individual patient, Theodorescu adds.
Choosing pancreatic cancer initially to study the validity of the platform was partly based on the very real issue that too many patients undergo treatment that may not be the best choice for their individual cancer. “Pancreatic cancer is very tough to treat, but headway is being made,” he explains. “Still today surgery is the only potential cure for the disease, yet many people don’t benefit from what is a very difficult procedure for the patient. We need a way to determine with very strong confidence who may benefit and who won’t benefit. And if a patient won’t benefit from the procedure, that patient needs to be urgently offered enrollment in a clinical trial, which may provide hope for longer survival.”
About the Molecular Twin Study
In the study, researchers performed the analysis using plasma and tumor tissue samples from 74 patients with stage I or stage II pancreatic cancer that could be surgically removed. They conducted “multi-omic profiling” of the tumor and the patient that included next-generation sequencing of DNA and whole-transcriptome RNA sequencing, tissue proteomics, plasma proteomics, lipidomics, computational pathology, and all standard patient demographics to generate individual clinical, genetic, and molecular data. The next step was to apply AI and machine learning approaches to this holistic data to determine what set of biomarker panels best predicted survival following surgery.
A model combining 6,363 different biological data points accurately predicted disease survival in 87 percent of patients. Going one step further, the team used AI to create a model of 589 data points that performed nearly as well. The findings were validated in independent datasets from The Cancer Genome Atlas, Massachusetts General Hospital (Boston), and Johns Hopkins University (Baltimore, Maryland).
Upon further investigation, the researchers showed that of all the profiling technologies used, evaluation of proteins found in the blood was the best single predictor of pancreatic cancer survival. “Genetic information is very helpful and very important in determining an individual patient’s risk of developing a cancer and we see that in pancreatic cancer,” Theodorescu notes. “But what we’re showing here is that its proteins may be the best way to determine whether a patient survives using a simple and cost-effective approach.”
A vital component in developing a better predictive model is not to look at cancer in isolation. Rather, Theodorescu has long hypothesized that the best predictive model would look at more than the tumor type and would have to incorporate information about the patient. “The cancer patient is not a cancer with legs. It’s a body that responds to an invader that is genetically different,” he says. “So I knew something was missing, something either on the tumor or on the host response to the tumor. To get the best predictive model we need more information from the cancer and more information from the host…to look at the problem in a holistic way. And that’s what the Molecular Twin Precision Oncology Platform gives us.”
Predictive models of cancer behavior or response to therapy can be improved because seemingly identical patients may have different outcomes, Theodorescu adds. “No two tumors are alike and no two patients are alike. What we did was take patients that had the same cancer type, which was pancreatic cancer, and who were at similar stages and had the same treatment. And what we did was show that looking at the cancer and host together, in a holistic way, will give you better predictions.”
In a research briefing accompanying the published study, Emma Lundberg, Ph.D., associate professor of bioengineering and pathology at Stanford University (Palo Alto, California) wrote: “The presented study demonstrates great potential for using multi-omics approaches in predicting therapeutic outcomes in pancreatic ductal adenocarcinoma. The authors have demonstrated that relying on CA 19-9 as a predictive marker for treatment outcomes is suboptimal. Instead, use of their predicted signatures may offer improved benefits in assessing the treatment efficacy. The study underscores the superiority of incorporating multi-omics data sources in predicting disease survival compared to relying solely on single-omic analyte input.”
Looking ahead, the Molecular Twin Precision Oncology Platform will be studied prospectively with larger cohorts of patients as well as in other cancers. “It’s AI, and this platform can be trained to predict practically all clinical outcomes and treatments,” Theodorescu says. “And since it can do that, the hope is that we can eventually be able to assign the patient to the very best initial treatment and then the very best subsequent treatments.”
The platform and its uses will continue to expand, Theodorescu explains. Investigators are adding data from additional patients and branching out to include additional types of data such as medical imaging and samples of the gut microbiome, among others.
He is hopeful about future uses for the technology. “To say I’m excited is kind of an understatement,” he says. “What we really want to do is create tests that can be used everywhere, not just in large academic medical centers. The world is a very big place, and not everyone has access to advanced resources and technologies. This platform can help bring precision oncology to everyone . . . to democratize the process.”