Man + Machine: Using Deep Learning for Early Detection of Pancreatic Cancer
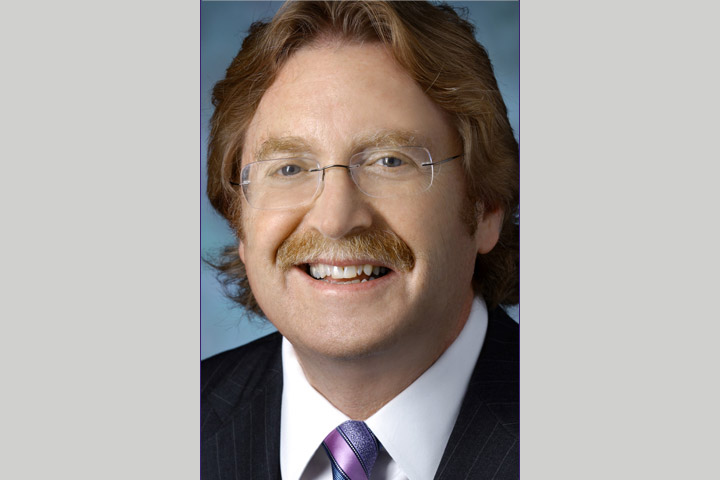
It wasn’t that long ago that so-called deep learning seemed the stuff of science fiction.
But now deep learning has become a big behind-the-scenes player in many aspects of our lives. It’s deep learning that allows a car to be driverless because it can distinguish between a fire hydrant, a stop sign, and an errant jaywalker. And what about voice control on our tablets or smart phones? Even movie recommendations on our streaming services use deep learning.
But deep learning is also poised to become an important player in our health care, especially in personalized medicine. And it may prove to be the answer to one of the most elusive goals in pancreatic cancer treatment: early detection.
Felix Felicis—The Felix Project
At Johns Hopkins, a group of radiologists, oncologists, geneticists, pathologists, computer scientists, computer students, and others are combining their various areas of expertise in a deep learning research study. It’s called the Felix Project. This multiyear, multimillion-dollar study funded by the Lustgarten Foundation is inspired by the Harry Potter series and the whimsical, magical potion called Felix Felicis. Take a sip, and you’ll have success in everything you do.
And, indeed, finding a way to detect the most common form of pancreatic cancer—pancreatic ductal adenocarcinoma (PDAC)—in its earliest stage, a window of time in which the only potentially curative option of surgery exists, would be counted among the greatest successes in a cancer that is considered almost uniformly fatal. The numbers are grim: PDAC is the third-leading cause of cancer deaths, and less than 20 percent of patients are eligible for surgery at the time of diagnosis. What these experts want to do is figure out whether deep learning algorithms can be developed to aid in the interpretation of MRI and CT scans of the pancreas. It’s these images that can signal early stage cancers.
Leading the team is noted radiologist Dr. Elliot K. Fishman, professor of radiology and oncology at the Johns Hopkins University School of Medicine, in Baltimore, Maryland. He likens the Felix Project to the Manhattan Project, the American-led effort to develop a functional atomic weapon before the Germans during World War II. “Sometimes projects only go so far in academia, and you may publish a little bit, but there’s no rush to get something done,” Fishman says. “But with (the Manhattan Project) there was a rush, an all-out effort to get something done. The same holds true with the Felix Project.”
That’s why for the past two years these experts have met every Wednesday, without fail, to see exactly how far they’ve come and what needs to be done. “We’ve made a commitment, and all of us involved in this project feel the same way,” Fishman says. “Our enemy is pancreatic cancer, and with this effort we can potentially save lives.”
Man + Machine = Deep Learning
Very simply, deep learning, which is a subset of AI, or artificial intelligence, teaches computers to do what we take for granted, which is to learn by example, whether that’s by images, text, or sound. Deep learning models are often startlingly accurate, often more so than humans. Computers are trained by using large sets of labeled data and what are called neural network architectures, which contain many different layers. “Basically, deep learning is an artificial intelligence function that imitates the way our brains process data and then from that data how we make decisions,” Fishman says. “There’s a lot more to it than putting a bunch of scans into a computer and hoping the computer does all the work. All the work that needs to be done needs to be done upfront by humans. Then the computer can take off and do what it does best, which is analyzing large amounts of data.”
To that end, the team of researchers has taught the computer to recognize the shape and architecture of the pancreas, which, by itself, was painstaking, since the shape of the pancreas can differ among patients. The next step is to teach the computer to recognize the differences between healthy pancreatic scans and those that show a pancreatic malignancy, even if those malignant changes are very subtle. So far, the team has tried out its algorithms with about 2,000 CT scans, including about 800 from patients with a confirmed diagnosis of pancreatic cancer, according to Fishman.
“At Johns Hopkins we have an incredible amount of pancreatic cancer data, but the scans all have to be annotated [labeled],” he says. “We have to show the computer all of the characteristics that make up a healthy pancreas and a diseased pancreas. It takes about four hours per scan to label a dataset.” The team also has to teach the model to recognize changes that may be benign, such as a pancreatic cyst, or a type of pancreatic malignancy called a neuroendocrine tumor.
But the effort seems to be worthwhile. So far, the algorithms that have been developed have about a 70 percent accuracy rate in detecting the pancreas and surrounding organs such as the liver. “That’s actually really good, and it will get better,” Fishman says. “You have to understand that sometimes it’s tough for a radiologist to find the pancreas.” Even better, deep learning accurately found PDAC with about 90 percent accuracy.
Fishman, as well as other experts, believe the use of deep learning and other forms of artificial intelligence will benefit not only patients but also the field of radiology. “This isn’t man against machine, because that’s a problem in which man always loses,” he says. “But when you have man with machine, our abilities get better.”
Fishman is understandably hopeful about the project. He sees thousands of pancreatic cancer scans yearly, and most are showing late stage disease. “It’s very frustrating because we know if it was found earlier, we could potentially offer a patient a cure. And I believe many of these cases could have been found earlier.”
With deep learning algorithms, he believes radiologists will be able to do more, with more accuracy. “The computer is quicker than us. It doesn’t get tired. It will find subtle changes the human eye may miss and say, ‘Hey, look here.’” Fishman adds, “Think about it, there are millions and millions of abdominal scans done every year. Add this algorithm, and you could have an early detection method for a terrible disease. Can you imagine what a difference that could make?”